COVID-19 Outlook: Lessons Learned from a Year of Modeling the COVID-19 Pandemic
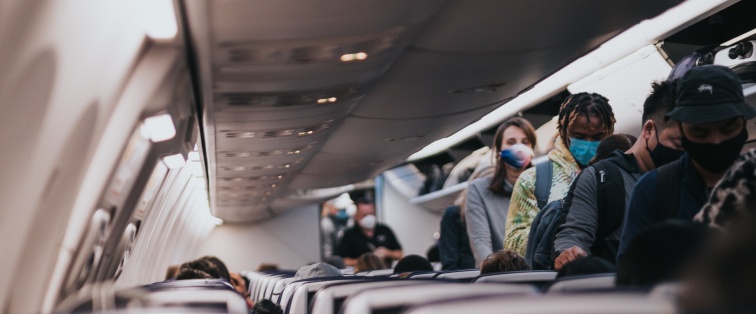
This week, with declining transmission and hospitalizations confirming that our worst days are behind us, our team is preparing to conclude the journey of PolicyLab’s county-level COVID-19 forecasting model, COVID-Lab, that began last March. Although our weekly updates will end next week, we will continue to monitor community transmission and may return from time to time to update the model. For example, circulating variants and undervaccination create uncertainty for next winter’s respiratory virus season, not just in the United States but globally. Should we identify emerging risk, we will share those findings. We also anticipate blogging at least once a month over the summer to update our guidance as communities prepare for next school year.
For now, here is this week’s updated national outlook based on our data:
- The national average test positivity rate dropped to 4.8% this week (down from 5.3%); Sixty percent of the 821 counties we follow in our model now have test positivity rates of 5%.
- One-third of the counties we follow had a higher test positivity rate this week compared to last week. The five states with an increased average test positivity rate include Arizona, Arkansas, Indiana, Louisiana and Wyoming—all states among the lowest in vaccination rates.
- Average reproduction numbers (a measure of transmission that estimates how many additional individuals, on average, will be infected by every positive case) again dropped below 1 to 0.92. However, one-quarter of counties still had reproduction numbers above 1, and 5% are demonstrating substantial transmission (reproduction numbers over 1.25). Cities in counties above 1.25 include Muncie, Ind., Shreveport and Baton Rouge, La., Chattanooga, Tenn., and Sioux, S.D.
- Nationally in the last month, adult hospital census declined 25% (still above 30,000 patients) and daily admissions declined 30%. Pediatric hospital census is falling at a slower pace, down only 10% in the last month, but is at a much lower volume (less than 1,000 children are currently hospitalized).
This week’s regional updates:
- Since April, there has been a 50-60% drop in adult hospital census in New York (from 5,100 to 2,400) and New Jersey (from 2,500 to 1,000), on top of the dramatic decline in case incidence we noted last week. Weekly case incidence in four of the five New York City boroughs is now below 100 cases per 100,000 individuals, and our projections show continued declines. These declines in case incidence and hospitalizations are in temporal response to rising vaccination rates.
- Improvements in Florida—both in declining transmission rates and in our model projections—are concentrated along the southeast coast near Miami and Broward County; this area has the highest vaccination rates across the state. Orlando and Tampa Bay are also improving, but their collar counties are not demonstrating the same rates of decline in transmission.
Above are the projections for Miami-Dade County in Florida.
- Hospitalization rates are rising, albeit slowly, in parts of the Southeast (Tennessee, Alabama and Mississippi), where vaccination rates are among the lowest in the country; only one-quarter of individuals in these states are fully vaccinated, and just over one-third are partially vaccinated.
- Counties in Indiana and West Virginia continue to see increased risk compared to other states in the region. For example, case incidence has been increasing in Indianapolis, and the city is now seeing more than 100 weekly cases per 100,000 individuals. Both states are also experiencing persistently elevated emergency department visits and hospitalizations. Notably in these states, under 40% of individuals are partially vaccinated and less than 30% are fully vaccinated.
Above are the projections for Marion County in Indiana.
- In California, increasing transmission persists in the Sacramento region, where vaccination rates are the lowest among the large metropolitan areas of the state.
- Hospitalizations have moved past peak in Oregon and Washington, as the region surpasses the 50% threshold of at least partial vaccination, with more than one-third of all residents in both states fully vaccinated. Our models project substantial declines in transmission across this area in the coming weeks.
Lessons Learned from a Year of Modeling the COVID-19 Pandemic
The famous economist John Maynard Keynes once quipped, “When the facts change, I change my mind. What do you do sir?” Throughout the last year, as we’ve witnessed a tragedy of more than 590,000 Americans losing their lives to COVID-19, the team behind COVID-Lab has felt fortunate to be able to provide real-time accounts of this pandemic down to the county-level. We hope the data we visualized helped to inform leaders as they furiously devised strategies to safely navigate their communities through this pandemic that has gripped our nation for over 15 months. We have learned much along the way, but our biggest lessons have been the importance of anchoring our guidance in data and being willing to adjust recommendations as new evidence emerges.
In PolicyLab’s 13-year history, our teams have consistently learned that local communities need to understand their own data in order to effect change. So, when the pandemic began in the U.S. in March 2020, we looked at national epidemic curves and projections and observed that while these data were helpful at a macro level, they had limited utility for individual communities. During the spring of 2020, as we watched case incidence grow rapidly in New York City, we knew that city’s experience would not necessarily be that of Philadelphia’s, where we lived. Similarly, Philadelphia’s experience might be vastly different than our neighbors in rural Pennsylvania or for many other communities across the country. At any given point in time, some areas might need to make difficult decisions to add public restrictions, while others might be able to navigate a different path to keeping businesses open and kids in school.
A local lens appeared to be critically important and was one that our team had the capacity to provide.
Thus began COVID-Lab, which, on a weekly basis over the last year, provided county-level four-week forecasts through a model that included 821 counties at its peak, representing 82% of the U.S. population across all 50 states and the District of Columbia. Our model is unique not only in its locally driven design, but also because it incorporated multiple behavioral and environmental factors that can influence transmission of the virus, beyond the innate properties of the virus itself.
However, this effort went beyond simply providing data for others to interpret. We elected to pen a weekly blog post to offer our interpretation of the data. The posts were products of a team intentionally assembled to include expertise in public health, infectious diseases, public policy, communications, epidemiology, statistics and data visualization. This collaboration provided a wide range of inputs on how best to navigate the pandemic given our model’s forecasts. With each week, we found our footing in predicting and then responding to the twists and turns of the pandemic. Importantly, we constantly challenged each other’s assumptions and innate biases. The data analyses and accompanying guidance offered in our weekly blog posts—whether related to public policy response or school safety plans—are the result of this deliberate, consensus-driven process executed by our interdisciplinary team.
We have learned many important lessons over the past year, but none more critical than the following:
Never be too sure of yourself.
One of the most difficult aspects of the past year was providing public guidance for a novel coronavirus with insufficient data, in the midst of an evolving pandemic and changing public attitudes and behaviors. How confident were we that our models were providing accurate forecasts? How much were children contributing to transmission? Were schools safe? Would we see another wave after the winter? The questions were innumerable.
To build and maintain the models, our team met at least twice weekly (often daily!) since last March, challenging ourselves to adapt a model that could remain reliable throughout the pandemic. The overarching goal of COVID-Lab was to be flexible enough to integrate multiple data elements, generative in nature to capture the dynamics of virus transmission and reasonably simple to be computed quickly. Our team took a unique approach in applying methods that had been used to understand the changing transmission dynamics of the Ebola virus to a hybrid statistical-epidemiological model that predicted local-level variation in COVID-19 transmission.
Built from the limited data available in spring 2020, COVID-Lab was iteratively validated and refined over the year, as data and knowledge of the virus accumulated. The result was a complex model that continuously incorporated data on local-area effects, seasonality, testing trends and social distancing measures. Our weekly forecasts allowed our team to offer counties and their leaders a sense of where they were at that moment and a glimpse of what was to come. Independent validation of our model against other national models, by a separate center within our health system, demonstrated superior performance—not perfect, but highly granular and accurate in seeing the fits and starts of a moving target.
A constant challenge for our team was to message the dynamic nature of the data we were producing. Of course, we all wanted recommendations that could be stated at the outset and would be long-lasting without much nuance. However, this was never in the cards with this pandemic. Our recommendations needed to remain flexible and change in response to emerging information. For example, we began the year—in the absence of strong data on school-based transmission at higher levels of community transmission—suggesting that schools await low levels of community transmission before resuming in-person education. However, it became clearer that schools’ safety protocols were effective in substantially reducing transmission risk in schools, even when the burden of disease in the community remained elevated. Recognizing this, we abandoned the absolutism of thresholds and pivoted to helping schools identify when linked in-school transmission was occurring.
Similar recalibration needed to occur when contact tracing was no longer revealing that restaurants or fitness centers that enforced strong safety protocols were meaningfully adding to community transmission. We advocated strongly from the fall onward that smart business reopening could occur throughout the pandemic. Finally, when many were overestimating the impact of variants on later stages of the pandemic, we followed the data and estimated that “a coming hurricane” in the spring of 2021 was not likely to happen. Each of these examples remind us that the data needed to be our North Star and we cannot base our estimates of what is to come on fear, speculation or hyperbole.
Beware of confirmation bias.
In the worst days of November and December, the fervor to return children to school at all costs became one of our greatest challenges. The desire to confirm what we wanted to see—that schools were safe under all conditions because children needed to be back in school—was the bias that most endangered our work during this critical time.
If there was consensus across all stakeholders—including public and private school leaders, school staff and their communities—it was that the negative impacts of the pandemic, particularly on those in lower-income communities, would continue the longer children remained out of school. And yet, emerging data on SARS-CoV-2 also revealed that while children were unlikely to develop severe COVID-19 illness, they were likely to contribute meaningfully to community transmission. The decision by United Kingdom officials to quickly shutter all of its schools after noting a dramatic increase in B.1.1.7 variant cases among school-age children was a reminder that children were an important source of transmission. Additional evidence of increased cases associated with youth sports leagues and surging rates among high school youth added to these concerns.
During this highly uncertain time, as hospitalizations and deaths were mounting across the U.S., we challenged ourselves to remain circumspect about the data. Yes, safety plans were helping many schools avert large outbreaks, but maintaining effective protocols would require steadfast commitments to these plans and, for schools that were able, testing of staff and high-risk students to reduce risk substantially from week to week. We ultimately recommended a short pause before the holidays, but quickly resumed planning for full in-school instruction with strong mitigation protocols after the new year and started developing, in partnership with local health departments and schools, one of the largest school-based testing programs in the country, Project: ACE-IT.
Take time to find common ground.
From a policy standpoint, we have been careful throughout the pandemic to help policymakers avoid an “all or nothing” approach—eliminating the virus entirely through lockdowns, business closures, or prolonged restrictions could be just as damaging an approach as pushing through toward herd immunity without any sensible restrictions like masking or reducing gathering size.
We have also encouraged policymakers and members of the public to remain open to new data that would challenge assumptions about uniform risk across regions, creating more flexibility, for example, to reopen businesses and schools more quickly in rural and suburban areas, so long as sensible safety plans and public masking requirements were in place. We provided the results and interpretation of our models to any leader interested in better understanding data relevant to their constituents. This included many discussions with local and state governments and with officials from the White House Coronavirus Task Force. We are hopeful that our data offered them an opportunity to conceive different strategies for balancing mitigation with economic and individual freedoms. Leaders valued this approach, as it signaled that we were not dug in to one set of recommendations and were open to a balanced plan that weighed competing risks. Likewise, we were impressed by the desire of many leaders to learn from our data and use this information to guide their decisions.
During these many conversations, we learned to become less prescriptive over time, recognizing that our role was to inform but not to place judgment on the decisions leaders made. Ultimately, we learned to value different opinions, amongst our teams, amongst community members, amongst the school leaders we were assisting, and amongst public health officials who were charged with the unenviable task of shaping plans for which consensus was never fully possible.
Looking back on the year, our team is humbled to have played a small part in a much larger story. While we sometimes drew the ire of those who rejected the science of the pandemic, as well as those who viewed prolonged public restrictions as the only calculus, we heard positive feedback from many more people looking for common sense approaches that permitted them room to navigate during a period of uncertainty. We achieved common ground more than we did not. At a time in which bitter divisions can be wedged against us, this most critical lesson, finding common ground even when we cannot all agree, is the one we hope to carry forward.
Jeffrey Gerber, MD, PhD, MSCE, is the associate director for inpatient research activities for Clinical Futures at Children’s Hospital of Philadelphia, and is a co-author of PolicyLab’s Policy Review: Evidence and Guidance for In-person Schooling during the COVID-19 Pandemic.